Non-parametric representation and prediction of single- and multi-shell diffusion-weighted MRI data using Gaussian processes
目的:dMRI有image distortion, signal loss, 等问题。为了校正这些问题,需要预测正确的dMRI图像应该是怎么样的。
Geostatistics: a class of statics that analyze and predict spatial/ spatiotemporal relationship
diffusion signal 在q-ball 上的性质
- The signal changes smoothly as the angle of the diffusion weighting direction changes.
- The signal is axially symmetric, i.e.the signal along g is identical to the signal along − g.
covariance function
covariance function 的来源:通过sample 一些图像,计算互相之间的covariance function,以对应角度差$\theta$作为自变量,画出函数图像,然后看看与怎样的convariance function 拟合的比较好。
- exponential model: $C(\theta)=e^{-\theta/a},\theta \in [0,\pi]$
- spherical model:
$\theta(\vec{g},\vec{g’})=arccos|<\vec{g},\vec{g’}>|$ (轴对称的性质)
Single-shell data
Optimize hyperparameters
marginal likelihood maximization: $log(p(\mathbf{y}|\beta,M))=-\frac{1}{2} \mathbf{y^TK_y^{-1}y}-\frac{1}{2}log|\mathbf{K_y}|+c$
$\mathbf{y}$是这个voxel上所有g 方向的signal 组成的向量。在所有的voxel $\mathbf{y}$上对上式求和。
其中$\beta$表示所有的hyperparameter,M表示选择的covariance function。
其中$K_y$是带有噪声项的covariance matrix。$K_y=K+\sigma^2I$
*用Laplace approximation来在两个covariance function中进行选择PRML中的6.4.6。(不是特别重要,GP 的预测效果和具体的covariance function 的具体形式关系不大)
用leave-one-out 尤其是cross validation的方法来检查hyperparameter估计效果
Multi-shell data
covariance function: $k(\mathbf{x,x’})=C_{\theta}C_b$,其中$C_{b}=exp(-\frac{(logb-logb’)^2}{2l^2})$。
两个b-value时的例子:

Optimization
梯度优化,PRML中的
启发式,本文中的Nelder–Mead simplex method
Results
这幅图表示的是一个voxel。三维图像上每个点的角度表示diffusion的角度,极径表示diffusitivity。红色的点表示测量值,灰色面表示GP prediction。
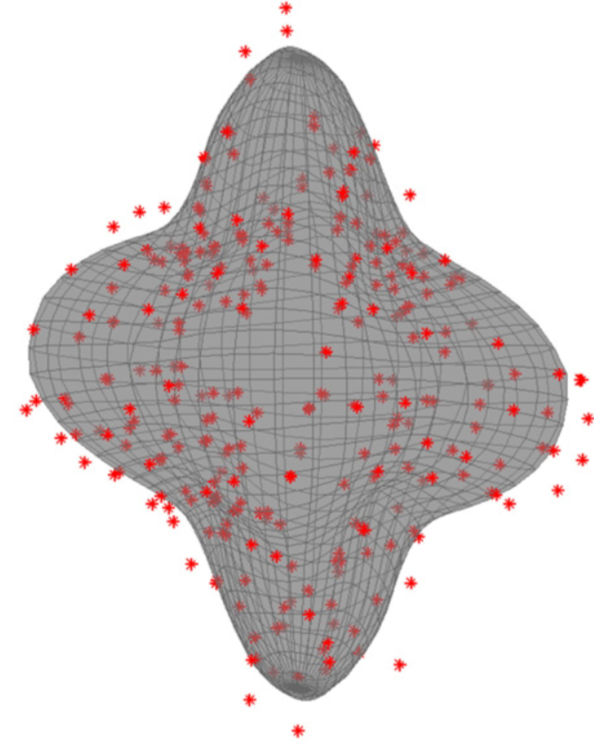